MRI Findings in Autism
Magnetic Resonance Imaging (MRI) has become an essential tool in understanding the neurobiological basis of autism spectrum disorder (ASD). By examining the brains of individuals with ASD, researchers have identified several abnormalities in MRI scans that provide valuable insights into the condition.
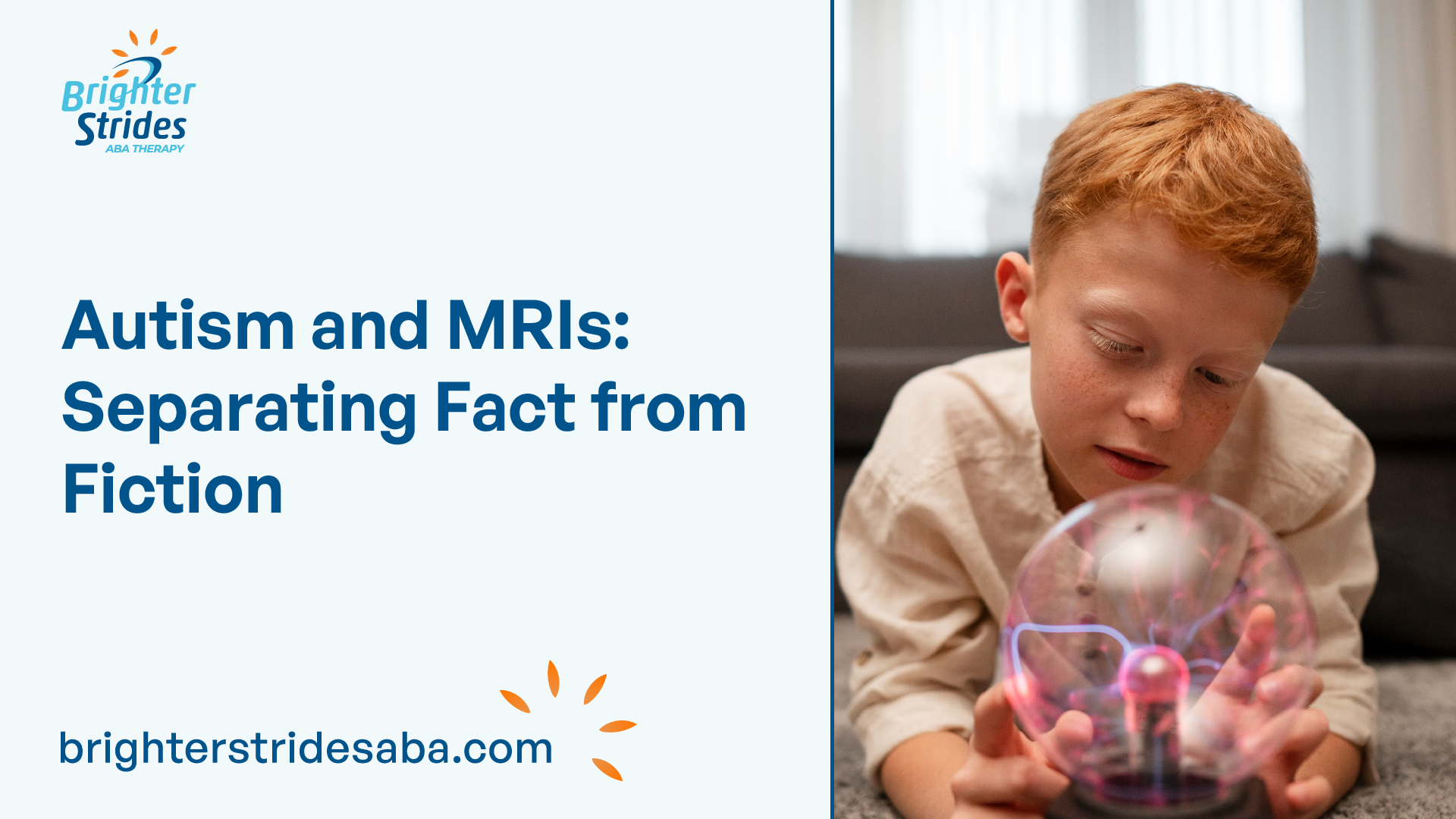
Abnormalities in MRI Scans
In a study of young children with ASD, routine MRI scans revealed abnormal findings in approximately 55% of the participants, with a higher prevalence in the high-functioning subgroup compared to typically developing children. These abnormalities included mega cisterna magna, ventricular anomalies, and abnormal white matter signal intensity. These findings suggest that there may be structural differences in the brains of individuals with ASD compared to those without the condition.
Role of MRI in ASD Diagnosis
MRI plays a significant role in the diagnostic process of ASD. It allows clinicians to visualize and analyze brain structures and networks associated with the condition. By using multiple MRI modalities, such as structural MRI (sMRI) and functional MRI (fMRI), researchers have been able to study brain changes in individuals with ASD.
It’s important to note that MRI findings alone cannot be used as a definitive diagnostic tool for ASD. The diagnosis of ASD is typically based on a comprehensive evaluation that includes clinical observations, medical history, and assessments by trained professionals. However, MRI findings can provide additional information that supports the diagnostic process.
It is worth mentioning that individuals with ASD may face challenges during MRI examinations due to sensory overload and limitations in the MRI environment. Effective communication, optimization and customization of the examination, and adjustments to the MRI environment are crucial for ensuring safe and successful MRI examinations for autistic individuals. However, barriers such as poor patient-radiographer communication, lack of specialized training for radiographers, and the unavailability of Special Educational Needs (SEN) experts can hinder the process.
While MRI findings provide valuable insights into the neurobiology of ASD, further research is needed to fully understand the relationship between these abnormalities and the complex nature of the condition. MRI, along with other diagnostic techniques, continues to contribute to the ongoing efforts to improve the early identification and understanding of autism spectrum disorder.
MRI Techniques for ASD
When it comes to studying autism spectrum disorder (ASD), MRI techniques have emerged as an efficient and noninvasive approach for clinical diagnosis and understanding the brain changes associated with ASD. Two commonly used MRI techniques in this context are structural MRI (sMRI) and functional MRI (fMRI).
Structural and Functional MRI
Structural MRI (sMRI) allows researchers and clinicians to examine the anatomical structures of the brain. It provides detailed images of the brain’s gray matter (GM), white matter (WM), and other structures. Through sMRI, studies have revealed alterations in brain structures in children with ASD, including differences in brain volume, cortical areas, amygdalae, hippocampus, and the posterior cranial fossa. These findings contribute to our understanding of the underlying neural differences in individuals with ASD.
Functional MRI (fMRI) goes beyond structural imaging and focuses on brain activity. By measuring changes in blood flow and oxygenation, fMRI provides insights into the functional connectivity and activation patterns within the brain. fMRI studies have allowed researchers to investigate the brain networks and functional abnormalities associated with ASD. These studies have helped identify brain regions involved in social cognition, language processing, and sensory integration, among others [2].
The combination of sMRI and fMRI techniques offers a comprehensive understanding of both the structural and functional aspects of the brain in individuals with ASD. These techniques provide valuable insights into the underlying neurobiology of ASD and contribute to the diagnostic process.
To ensure the successful implementation of MRI techniques for individuals with ASD, it is important to address the challenges they may face in the MRI environment. Autistic individuals often experience sensory overload and may have difficulties with communication and adaptation to the MRI environment. Effective communication, customization of the MRI examination, and adjustments to the MRI environment are essential for safe and effective MRI examinations for individuals with ASD. Improving patient-radiographer communication, providing access to Special Educational Needs (SEN) experts, and offering specialized radiographer training can help overcome these challenges and enhance the overall MRI experience for individuals with ASD.
MRI techniques have proven to be valuable tools in studying ASD, providing valuable insights into the structural and functional aspects of the brain. By utilizing these techniques, researchers and clinicians can continue to advance our understanding of ASD and contribute to improved diagnostic methods and interventions.
Machine Learning in ASD Diagnosis
Advancements in machine learning techniques have shown promise in aiding the diagnosis of autism spectrum disorder (ASD) using MRI scans. Two notable approaches in this field are the Computer-Aided Diagnostic (CAD) system and the discriminative model for ASD.
Computer-Aided Diagnostic System
The CAD system is a proposed method for diagnosing individuals with ASD using structural MRI scans. This system aims to identify morphological anomalies within specific brain regions of ASD subjects. The CAD system achieves an average balanced accuracy score of 97±2% on the Autism Brain Imaging Data Exchange (ABIDE I) dataset, as stated in a study.
By utilizing machine learning algorithms, the CAD system can analyze the structural features extracted from MRI volumes. These features are then compared to a reference database to identify deviations associated with ASD. The high accuracy achieved by the CAD system demonstrates its potential as a valuable tool in the diagnosis of ASD.
Discriminative Model for ASD
Another approach in machine learning for ASD diagnosis involves creating a discriminative model using morphological features extracted from structural MRI (sMRI) volumes. This model aims to accurately classify individuals as either having ASD or being typically developed (TD).
The discriminative model utilizes a machine-learning approach, leveraging the power of algorithms to identify patterns and distinguish between ASD and TD subjects. The proposed pipeline achieves high accuracy in classifying ASD subjects, both locally and globally. The local model achieves a balanced accuracy score above 0.65 for most sites, while the global model achieves a balanced accuracy score of 71.6%±2.4%.
To create the discriminative model, local neuro-atlases are generated for each site using the Recursive Feature Elimination with Cross-Validation (RFECV) algorithm and selected morphological features. These neuro-atlases represent the brain regions and imaging markers associated with ASD. By comparing the features selected by the local models, common features can be identified and used in the global model.
The pipeline incorporating the discriminative model has demonstrated improved accuracy in classifying ASD subjects compared to previous studies. In comparison to a study by Katuwal et al., the proposed pipeline exhibits better performance in accurately diagnosing ASD using MRI data.
Machine learning techniques, such as the CAD system and the discriminative model, hold significant potential in improving the accuracy and efficiency of ASD diagnosis through the analysis of MRI scans. These advancements contribute to the ongoing efforts to better understand and diagnose autism spectrum disorder.
Neuroimaging in Autism
Neuroimaging techniques, such as magnetic resonance imaging (MRI), have provided valuable insights into the neurological underpinnings of autism spectrum disorder (ASD). By examining the brain structures and functions of individuals with ASD, researchers have identified anomalies and alterations that contribute to our understanding of this complex condition.
Anomalies in Brain Structures
Studies utilizing structural MRI have revealed anomalies in various brain structures in individuals with ASD. These anomalies include differences in brain volume, cortical areas, amygdalae, hippocampus, and the posterior cranial fossa. These findings suggest that there are significant differences in the size and shape of certain brain regions in individuals with ASD compared to neurotypical individuals.
Alterations in Gray and White Matter
Research has also demonstrated alterations in gray matter (GM) and white matter (WM) in the brains of individuals with ASD. Structural MRI studies have reported differences in GM and WM between ASD and control groups. These alterations may affect the connectivity and communication between different brain regions, potentially contributing to the cognitive and behavioral characteristics associated with ASD.
To better understand the specific alterations in GM and WM, advanced neuroimaging techniques, such as diffusion tensor imaging (DTI), have been employed. DTI allows researchers to examine the integrity and organization of WM tracts in the brain. Studies using DTI have identified abnormalities in WM tracts that connect different brain regions in individuals with ASD, further highlighting the importance of these structural alterations in the neurobiology of ASD.
By utilizing neuroimaging techniques like MRI, researchers continue to uncover valuable information about the structural and functional differences in the brains of individuals with ASD. These findings contribute to our understanding of the underlying mechanisms of ASD and may help improve diagnostic processes, treatment approaches, and interventions for individuals on the autism spectrum.
Diagnostic Techniques for Autism
When it comes to diagnosing autism spectrum disorder (ASD), various techniques have been developed to aid in the identification and assessment of individuals with ASD. In this section, we will explore two diagnostic techniques: facial analysis for diagnosis and edge computing in autism diagnosis.
Facial Analysis for Diagnosis
Facial attributes play a significant role in diagnosing autism. Researchers have found that facial expressions, eye contact, and body language can provide valuable insights into the presence of ASD. Machine learning algorithms are being developed to detect subtle differences in facial attributes that may indicate ASD, aiding in the early identification of children who may benefit from appropriate interventions.
By utilizing machine learning algorithms, these systems can analyze facial images and detect patterns associated with ASD. For instance, a proposed model based on deep learning using a transfer learning strategy achieved an impressive accuracy rate of 92.18% for autism recognition from facial images of children. This model demonstrates low computational complexity and is suitable for resource-constrained devices like smartphones [5].
Facial analysis for diagnosis holds great potential in educational environments, where it can assist in identifying ASDs. A model based on the AlexNet architecture for machine learning, combined with transfer learning, has shown high efficacy in using face analysis for autism identification in a school setting. This technique enables early detection and intervention, promoting better outcomes for individuals with ASD.
Edge Computing in Autism Diagnosis
Edge computing is emerging as a promising approach for autism diagnosis. This technique involves analyzing large volumes of data in real-time, providing accurate and reliable results for the diagnosis and treatment of ASD. Additionally, edge computing can help track the progress of patients over time and automate the process of collecting and analyzing data for autism surveillance.
By utilizing edge computing, clinicians and researchers can perform complex analyses closer to the source of data generation. This approach reduces latency and ensures real-time processing, which is crucial for timely diagnosis and intervention. Edge computing can be particularly valuable in situations where internet connectivity may be limited, such as in remote areas or resource-constrained settings.
The use of edge computing in autism diagnosis allows for the integration of various data sources, including medical records, wearable devices, and sensor data. This comprehensive approach enables a more holistic understanding of an individual’s condition, facilitating personalized treatment plans and ongoing monitoring of progress.
By harnessing the power of facial analysis and edge computing, healthcare professionals and researchers can advance the field of autism diagnosis, leading to earlier identification and intervention for individuals with ASD. These techniques offer the potential for improved outcomes and a better understanding of the unique needs of individuals on the autism spectrum.
Machine Learning in Cognitive Neuroscience
Machine learning techniques have significantly contributed to the field of cognitive neuroscience, particularly in studying autism spectrum disorder (ASD) and understanding its neurophysiological basis. In recent years, these methods have been employed to analyze fMRI (functional magnetic resonance imaging) data from individuals with autism and typical controls, yielding classification accuracies ranging from 48.3% to 97%.
fMRI Data Analysis
fMRI data analysis plays a crucial role in utilizing machine learning algorithms to study ASD. Resting-state functional connectivity (FC) measures have emerged as potential diagnostic biomarkers for ASD. Studies have consistently identified alterations in FCs between specific brain regions, including the posterior cingulate cortex (PCC), thalamus, and default mode network (DMN), when comparing individuals with ASD to typical controls.
Task-based fMRI data has also proven valuable in achieving higher classification accuracies compared to resting-state fMRI data. However, the limited availability of large datasets for task-based fMRI poses challenges for generalization and broader application.
Feature Selection Methods
Feature selection methods play a crucial role in enhancing the accuracy of machine learning models by reducing the dimensionality of features. By selecting the most informative features, these methods improve classification accuracy and efficiency. Studies incorporating feature selection methods have consistently demonstrated higher accuracies compared to those without feature selection.
The choice of atlas used for constructing FC features can also impact classification accuracy. The Automated Anatomical Labeling (AAL) atlas is commonly utilized and has consistently identified informative brain regions and networks, including the DMN. The selection of an appropriate atlas is crucial for ensuring accurate and reliable feature construction in fMRI data analysis.
In the field of cognitive neuroscience, machine learning techniques provide valuable insights into the neurophysiological aspects of autism. By leveraging fMRI data analysis and employing feature selection methods, researchers can identify informative brain regions, networks, and connectivity patterns associated with ASD. These advancements contribute to a deeper understanding of autism and hold promise for improving diagnostic approaches and treatment strategies.
References
- https://www.ncbi.nlm.nih.gov/pmc/articles/PMC7602717/
- https://www.ncbi.nlm.nih.gov/pmc/articles/PMC9410622/
- https://www.sciencedirect.com/science/article/abs/pii/S1078817421001243
- https://www.ncbi.nlm.nih.gov/pmc/articles/PMC8774643/
- https://journalofcloudcomputing.springeropen.com/articles/10.1186/s13677-023-00558-9
- https://www.frontiersin.org/journals/neuroscience/articles/10.3389/fnins.2021.697870/full